Testing the Profitability of Football Power Ratings
Posted 5th October 2015
In his book Profitable Football Betting (2002), Paul Steele describes 15 different match rating systems one can use to help predict the outcome of football matches. Testing over 5 seasons of data (1997/98 to 2001/02) from the English and Scottish divisions, Paul ranked the systems according their top 700 predictions. The best system was his "Power Ratings" method. In this article I have set out to test whether these Power Ratings can actually generate a profit.
A rating system provides a quantitative measure of the superiority of one team over their opposition. Such superiority is determined by analysing and comparing one or more aspects of past performance for each of the sides. Power Ratings attempt to take into account both the quality of the opposition a team faces as well as the number of goals they score in each match. Every team starts a new season with 10 points. After each match Paul Steele calculates the new Power Ratings as follows:
Rating change for home team = [goal difference - ratings difference - home advantage)] x Adjuster
This is then added to the original home team rating.
The rating change for the away team is simply the negative for that of the home team.
The adjuster is the "machine" around which the Power ratings methodology operates. Paul Steele tested a number of different values and found that 0.25 was the best one. The home advantage is equivalent to twice the adjuster, so in this case 0.5
Let's look at an example. If Liverpool, with a rating of 11, play Watford with a rating of 9 and win 5-0, the ratings change for Liverpool will be:
[(5 - 0) - (11 - 9) - 0.5] x 0.25 = 0.625
Liverpool's new rating would be 11.625, whilst Watford would fall to 8.375.
Suppose instead Liverpool won 1-0. This time their rating would fall to 10.625, whilst Watford's would rise to 9.375. Although Watford lost the game, they were playing a much higher rated team and were playing away, such that a 1-0 defeat would be considered slightly better than par.
One might argue that an inherent weakness of this methodology is that all teams are equally rated at the start of the season. This is certainly true, but the adjuster ensures that ratings quickly equilibrate to meaningful values after only a few matches. Nevertheless, in testing the profitability of the Power Ratings I omitted the first 5 games played by any team.
To test the profitability of any match rating we need to turn them into probability estimates for result outcomes. I have previously described how this might be done by means of a simple linear regression in my Football Betting Advice Guide. A more detailed version is available in my first book Fixed Odds Sports Betting and also in my Football Ratings PDF document.
Match ratings for every professional English football league match during the seasons 2010/11 to 2014/15 were calculated and then ranked in ascending order. The regression charts below have been produced by computing 250-point running averages for the ratings and actual results alike, from which regression equations haven been estimated. These equations essentially allow one to convert a match rating into an estimated outcome probability. From this we can then theoretically identify value bets, where the Power Ratings method estimates a win to be more likely than does the bookmaker.
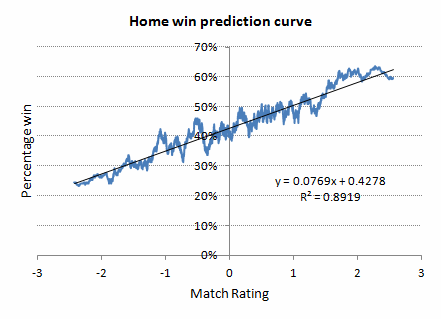

Ignoring the first 5 games of the season, the profit time series below shows how betting home and away value bets as indentified by this methodology (9,316 bets) compares to a blind betting of all home and away outcomes (18,042 bets). Odds were best market prices. Draws have been ignored since it's not clear to me how they are really anything other than random. [We might argue that home and away wins are as well, as I tried to do preveiously.]

Evidently there is not a whole lot of difference. Blind betting returned a yield of 0.37%, compared to 0.33% from the Power Ratings. Conceivably, betting over half of all possible outcomes is not selective enough. What if we increase the margin before which we choose to place a bet? The table below shows how doing so reduces the number of bets we place and increases the yield we see. Unfortunately, however, the average odds we are betting lengthen, predictably because increasing the value margin eliminates a disproportionate number of shorter prices. As average odds increase, so does the influence of luck, or speaking more statistically, variance. By the time we are betting at average odds greater than 10 we are getting some pretty healthy yields, but testing them for significance (the p-value) shows that they really aren't anything we could rightfully call representative of predictive skill (p-value < 0.05). The likelihood is that as we become more selective in our choice of value, we have just become a bit luckier.
Value
ratio |
Bets |
Avg odds |
Yield |
p-value |
1 |
9316 |
4.10 |
0.33% |
0.43 |
1.01 |
9037 |
4.13 |
0.35% |
0.42 |
1.02 |
8746 |
4.18 |
0.73% |
0.35 |
1.03 |
8449 |
4.22 |
0.77% |
0.35 |
1.04 |
8172 |
4.27 |
1.19% |
0.28 |
1.05 |
7911 |
4.31 |
0.93% |
0.33 |
1.06 |
7655 |
4.36 |
1.10% |
0.30 |
1.07 |
7380 |
4.41 |
1.16% |
0.30 |
1.08 |
7101 |
4.47 |
0.69% |
0.38 |
1.09 |
6860 |
4.51 |
0.39% |
0.43 |
1.1 |
6613 |
4.57 |
0.47% |
0.42 |
1.125 |
6078 |
4.69 |
0.35% |
0.44 |
1.15 |
5574 |
4.82 |
0.90% |
0.37 |
1.175 |
5088 |
4.97 |
0.73% |
0.40 |
1.2 |
4682 |
5.11 |
0.25% |
0.47 |
1.25 |
3923 |
5.41 |
0.12% |
0.49 |
1.3 |
3306 |
5.72 |
1.37% |
0.36 |
1.35 |
2786 |
6.05 |
2.29% |
0.30 |
1.4 |
2352 |
6.41 |
3.72% |
0.22 |
1.45 |
2001 |
6.77 |
4.62% |
0.20 |
1.5 |
1745 |
7.11 |
4.79% |
0.21 |
1.6 |
1322 |
7.80 |
6.10% |
0.20 |
1.7 |
1022 |
8.53 |
6.49% |
0.23 |
1.8 |
826 |
9.16 |
8.05% |
0.22 |
1.9 |
684 |
9.76 |
12.87% |
0.14 |
2 |
564 |
10.36 |
17.35% |
0.11 |
2.25 |
365 |
11.81 |
19.25% |
0.15 |
2.5 |
249 |
13.03 |
14.25% |
0.27 |
2.75 |
177 |
14.31 |
32.25% |
0.15 |
3 |
133 |
15.46 |
36.33% |
0.17 |
In general it has to be argued that Power Ratings do a good job of replicating the bookmakers odds but no more. When Paul Steele first tested his Power Ratings for predictability (seasons 1994/95 to 1998/99) his top 700 home and away predictions showed a success rate of 73% and 55% respectively. A clue as to how lucky this might have been can be found in comparing these figures to those he subsequently found for the 1997/98 to 2001/02 seasons which he published in his book. These had fallen to 67% and 46%. My top 700 predictions had success rates of 60% and 44%. The question has to be asked: why choose the top 700? Is there something significant about that number or was this just a certain set of matches that produced healthy looking prediction percentages? If the latter, this sort of data mining will lead you towards all sorts of spurious conclusions.
|